April 25, 2025 • Nelson Cicchitto
The Role of Identity Management in Securing Artificial Intelligence Systems: A Zero-Trust Approach
Discover how advanced identity management solutions protect AI systems through zero-trust principles, and adaptive authentication
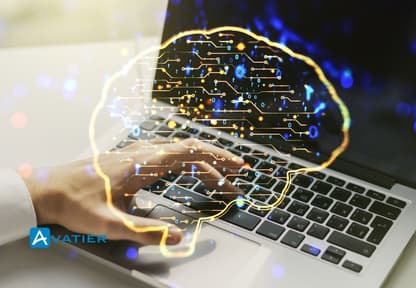
As artificial intelligence increasingly permeates enterprise systems, a critical security gap is emerging: traditional security measures weren’t designed for an AI-first world. With organizations implementing AI at unprecedented rates—Gartner predicts that by 2025, 80% of enterprises will have deployed AI orchestration platforms—the question of who (or what) can access sensitive systems has never been more complex.
Identity management isn’t just about human users anymore. It now encompasses machine identities, AI agents, and the complex web of permissions required when automated systems make decisions with minimal human oversight. The security risks are substantial: according to IBM’s 2023 Cost of a Data Breach Report, organizations with immature identity and access management were breached at more than twice the rate as those with advanced programs, with breaches costing an average of $4.35M.
Why AI Systems Require Specialized Identity Management
Traditional identity management was designed for predictable human access patterns. AI systems, however, present unique challenges:
- Machine Identity Proliferation: Machine identities (including AI agents) now outnumber human identities by 45:1 in the average enterprise, according to CyberArk research.
- Rapid Access Requirements: AI systems may require immediate, dynamic access to multiple data sources simultaneously.
- Privileged Access Concerns: AI often needs elevated access rights to perform advanced functions, creating potential security vulnerabilities.
- Audit Trail Complexity: Tracking exactly what AI systems access and why presents significant challenges for compliance and security teams.
These factors demand a reimagined approach to identity management—one that can secure both human and machine interactions with sensitive data and systems.
Zero-Trust: The Foundation for AI Security
The zero-trust principle—”never trust, always verify”—becomes even more crucial in AI environments. Unlike human users who might access systems during business hours from predictable locations, AI systems often operate continuously, accessing data across multiple security boundaries.
According to Avatier’s security framework, a zero-trust approach to AI security requires:
- Real-time authorization verification for every system interaction
- Contextual analysis of access requests from both humans and machines
- Fine-grained access controls based on need-to-know principles
- Continuous monitoring of all system operations
This comprehensive approach dramatically reduces the attack surface available to threat actors targeting AI systems. By implementing identity-centered controls at every level, organizations can allow AI innovation while maintaining robust security guardrails.
The Four Pillars of AI Identity Security
A comprehensive identity management strategy for AI systems rests on four fundamental pillars:
1. Machine Identity Governance
Just as human identities require lifecycle management, machine identities—including AI agents, service accounts, and automated processes—require robust governance controls. According to Gartner, by 2025, 60% of organizations will have experienced a major security breach related to unmanaged machine identities, certificates, and secrets.
Machine identity governance must address:
- Automatic provisioning and deprovisioning of machine identities
- Credential rotation and secrets management
- Just-in-time access to sensitive resources
- Detailed audit trails of all machine identity activities
Avatier’s Identity Anywhere Lifecycle Management provides the foundation for comprehensive machine identity governance, enabling organizations to manage the complete lifecycle of both human and non-human identities from a unified platform.
2. Dynamic Access Controls for AI Systems
Traditional role-based access control (RBAC) proves insufficient for the dynamic nature of AI operations. Modern AI systems require Attribute-Based Access Control (ABAC) or more sophisticated models that can make real-time decisions based on multiple factors:
- Data sensitivity classification
- AI operation type
- Time and context of access request
- Risk score of the requested operation
- Previous access patterns and anomaly detection
These dynamic controls ensure AI systems can access exactly what they need—nothing more, nothing less—reducing the potential attack surface.
3. Continuous Authentication and Authorization
For AI systems, authentication can’t be a one-time event. Modern identity management platforms must continuously validate that AI operations remain within approved parameters. This includes:
- Real-time monitoring of API access patterns
- Behavioral analysis to detect anomalous AI operations
- Step-up authentication for high-risk operations
- Automatic session termination when abnormal patterns emerge
According to an Okta study, organizations implementing continuous authentication technologies reduced credential-based attacks by 79% compared to those using periodic authentication methods.
4. Centralized Auditing and Compliance
AI systems often operate across regulatory boundaries, accessing data governed by different compliance regimes. Centralized auditing becomes essential to maintain visibility and demonstrate compliance.
Avatier’s Access Governance solutions enable organizations to:
- Maintain comprehensive audit trails of all AI system operations
- Automatically generate compliance reports for AI data access
- Detect potential compliance violations in real-time
- Provide evidence of security controls for AI operations
These capabilities help enterprises satisfy increasingly stringent regulatory requirements around automated decision-making systems while maintaining operational efficiency.
Implementing AI-Ready Identity Management: A Practical Framework
Transitioning to an AI-ready identity management framework requires a strategic approach focused on both immediate security needs and future adaptability.
Phase 1: Identity Foundation
Start with establishing a solid identity foundation that includes:
- Comprehensive inventory of all machine identities and AI systems
- Centralized identity repository that includes both human and machine identities
- Automated lifecycle management for all identity types
- Baseline access policies for AI systems
Phase 2: Zero-Trust Implementation
Build zero-trust architecture elements specifically for AI interactions:
- Implement least-privilege access for all AI components
- Deploy continuous verification mechanisms
- Establish network segmentation for AI workloads
- Create monitoring capabilities for all AI-to-data interactions
Phase 3: Advanced Governance
Develop sophisticated governance capabilities:
- Implement AI-specific access certification processes
- Create separation of duties for critical AI functions
- Deploy anomaly detection for AI behavior
- Establish remediation workflows for potential violations
Phase 4: Continuous Improvement
Maintain an evolving security posture:
- Regular security assessments of AI systems
- Threat modeling for new AI implementations
- Adaptation of security controls based on emerging threats
- Ongoing training for security personnel on AI-specific risks
The Future of AI Identity Management
As AI systems become more autonomous, identity management must evolve to address increasingly complex security scenarios. Several emerging trends will shape this evolution:
AI-to-AI Authentication
As AI systems interact with other AI systems, robust machine-to-machine authentication becomes critical. SailPoint predicts that by 2026, over 70% of enterprise data flows will involve at least one AI-to-AI interaction, requiring specialized identity protocols.
Federated AI Identity
Organizations will need to manage AI identities across organizational boundaries. This requires sophisticated federation protocols and trust frameworks specifically designed for machine identities.
Intent-Based Authorization
Beyond attribute-based access, future systems will evaluate the intent of AI operations, determining if requested actions align with approved AI missions and goals.
Quantum-Resistant Identity
As quantum computing threatens traditional cryptography, AI identity systems must implement quantum-resistant algorithms to secure authentication and authorization processes.
Conclusion: Identity as the Foundation of AI Security
As organizations accelerate AI adoption, identity management has emerged as the critical foundation for secure AI operations. The traditional security perimeter has dissolved, replaced by identity-centered security that must govern both human and machine access to sensitive resources.
Implementing a comprehensive identity strategy for AI systems isn’t merely a security best practice—it’s a business imperative. Organizations that fail to secure their AI systems face not only breach risks but also regulatory penalties, reputational damage, and potential liability for autonomous system actions.
By implementing zero-trust principles, organizations can secure their AI investments while enabling the innovation these powerful technologies promise. Advanced identity management solutions like those from Avatier provide the foundation for this new security paradigm, enabling organizations to confidently deploy AI while maintaining robust security and compliance.
As the boundary between human and machine operations continues to blur, identity management will remain the critical security control that defines what each entity—human or AI—can access, when, and under what circumstances. Organizations that master this discipline will be positioned to leverage AI’s full potential while maintaining the trust of customers, partners, and regulators.
The future of AI security isn’t about building higher walls—it’s about knowing precisely who and what is accessing your systems, and ensuring that every interaction follows established security principles. Identity management isn’t just part of AI security—it is the foundation upon which all other security controls depend.